Effect of the Number of Maxima and Stimulation Rate on Phoneme Perception Patterns Using Cochlear Implant Simulation
Article information
Abstract
Purpose
Maximizing speech perception for cochlear implant (CI) users can be achieved by adjusting mapping parameters. The object of this study was to investigate optimal sets of parameters of stimulation rate and the number of maxima in the CI system.
Methods
Listeners’ consonant and vowel perception was measured for different combinations of the number of maxima and stimulation rate using cochlear implant simulated stimuli. Twelve sets of speech stimuli were systematically created by changing the number of maxima and stimulus rate and were presented to 18 listeners with normal hearing.
Results
The group mean percent correct scores indicated only two pairs of parameter combinations showed significantly different results. A rate of 1,800 pps and 6 maxima resulted in significantly better consonant performance compared to a rate of 500 pps and 20 maxima. In addition, the 900 pps/8 maxima condition was significantly better compared to 500 pps/20 maxima for the vowel test. Analysis of listeners’ confusion patterns revealed they were more likely to make perception errors for the consonants /ð/, /l/, and /r/ and for the vowels /∧/, /e/, /æ/, and /ɛ/. Information transmission analysis indicated that, among other features, the voicing feature was transmitted best for consonant recognition and the backness feature was the most transmitted for vowel recognition.
Conclusions
The results of this study using vocoded speech with listeners with normal hearing contribute to a better understanding of CI users’ confusion patterns and possible ways to optimize cochlear implant signal processing strategies.
INTRODUCTION
Over the past few decades, cochlear implants (CIs) have become an alternate solution to hearing aids for people with severe-to-profound sensorineural hearing loss. Despite scientists’ continuous efforts to facilitate hearing ability, however, there are still unresolved issues that CI users’ experience (e.g., fine speech perception, speech perception in noise, and music perception).
When CI patients complain about these issues, one of the primary methods audiologists can employ is to adjust the mapping parameters to resolve their perceptual complaints. Numerous investigations have studied changes in signal processing strategies and mapping parameters, and results have shown corresponding variance in CI users’ speech perception performance [1–12]. However, to date, no single set of mapping parameter adjustments has produced the optimal solution for CI patients due to a vast amount of confounding patient variables and mixed results from studies.
A large number of studies [13–23] have investigated consonant and vowel perception ability for CI listeners and have used several statistical methods to systematically analyze results from such phoneme recognition tests. In phoneme recognition tests, listeners are asked to identify an individual target segment (i.e., a consonant or vowel) presented in a given particular format of nonsense syllable. Phoneme recognition tests have some disadvantages because they miss the rapid nature of the speech stream and do not take advantage of contextual effects [24]. However, since phonemes are the smallest units in speech structure specified as bundles of distinctive features, they can provide phonological cues that can be beneficial for speech recognition studies. Distinctive features are basic units corresponding to a particular phonological or articulatory property in phonemes. Since the first appearance of Distinctive Feature Theory [25], there have been continuous attempts to refine such features [26–29], and results from these studies have been applied to phoneme recognition tasks to investigate speech perception patterns with regard to phonological and articulatory features. Confusion matrices in phoneme recognition studies arrange listeners’ responses in rows as a function of presented stimuli in columns. In the matrix, each entry is grouped based on its articulatory or phonological features, and groups of entries are partitioned again corresponding to their sub-distinctive features [30]. This series of procedures not only improves the efficiency of identifying error patterns, but also could form the basis for quantifying information for further analyses. The results of phoneme recognition can be further analyzed using Information transfer (IT) analysis introduced by Miller and Nicely (1955). The IT measures the percent of speech information transmitted to listeners with regard to the distinctive features of phonemes.
CI signal processing
CIs use well-researched signal processing strategies to encode the incoming speech signal for the listener. Of the several signal processing techniques available, n-of-m type strategies selectively emphasize spectral information by focusing on the number of channels that contain the highest amplitudes in the signal at any one point in time [31]. The underlying assumption of an n-of-m strategy is that the frequency bands containing the highest energies have the most important information. Incoming acoustic energies are distributed into corresponding frequency channels as a filter-bank, envelope amplitudes are estimated, and then the electrodes that correspond to the channels with the highest amplitudes are activated. Thus, the dominant channels (n) out of the entire number of channels (m) are stimulated in real time. The advantages of this technique are reduced channel interaction and prolonged battery life.
Maxima
The activated channels in the n-of-m speech processing strategy are called maxima. Commercial speech coding strategies requiring adjustment of the number of maxima are Cochlear Corporation’s Spectral Peak (SPEAK) and Advanced Combination Encoder (ACE) strategies [31,32]. Although these two strategies are similar in the way they deliver the signal, ACE has advantages over SPEAK by providing higher rates of stimulation and wider spectral ranges. The SPEAK strategy divides the frequency range of input speech into 20 programmable bandpass filters, and the 6 to 10 frequency bands that contain the greatest energy are selected. The ACE strategy, in contrast, allows up to 22 spectral maxima which include the greatest energy. Only a few studies exploring the effect of the number of maxima on speech recognition have been completed to date. In a clinical study using CI simulation by Dorman et al. (2002), speech recognition performance was compared using a fixed-channel versus a channel-picking strategy. In quiet conditions, the fixed-channel strategy required 8 channels of information to reach maximum speech recognition compared to only 6 maxima out of 20 (6-of-20) for the channel-picking strategy. In noise, maximal speech understanding was achieved by a channel-picking strategy with 9 maxima out of 20 channels (9-of-20) compared to a fixed-channel strategy with 10 channels. More relevant to the goal of the present study, Plant et al. (2002) examined speech perception outcomes for eight Nucleus 24 CI patients with stimulus rates fixed at 900 pulses per second (pps) per channel (ch), and the number of maxima was varied from 6, 8, 12, and 16 using the ACE strategy. Based on their results of speech recognition and subject preferences, they recommended 8 or 12 maxima in the ACE strategy for improved performance. These studies, however, do not account for the detailed interaction effects that can occur when both stimulation rate and number of maxima are varied. Thus, supplemental studies are needed to determine whether this number of 8 to 12 maxima is sufficient for the latest signal processing technologies and whether other parameters may have an influence on the results.
Stimulation tate
Another critical parameter of signal processing strategies is stimulation rate as it plays an important role in transferring temporal cues from rapidly changing speech signals. The per-channel stimulation rate in a cochlear implant is the number of pps delivered to individual electrodes, whereas the total stimulation rate (TSR) is the number of pulses per second of all of the channels in the electrode array. The TSR can be calculated by multiplying the per-channel stimulation rate and the number of channels. CI systems vary in the range of stimulation rates available. They range from low (<500 pps/ch), to moderate (500–1,000 pps/ch), to high (>1,000 pps/ch) depending on the type of device and processing strategy used (1). There have been a number of studies that have attempted to determine the relationship between stimulus rate and speech perception performance, but clear conclusions have not been made to date due to mixed results from different studies [1,3,5,8,9,11,12].
Some studies have provided empirical evidence supporting the benefits of increasing electrical stimulation rate [3,8,9]. Generally acceptable rationales behind the advantages of high stimulation rates are stochastic firing, improved temporal sampling, expanded dynamic range and lower thresholds [11]. Loizou et al. (2000) studied the effect of parameter variations on speech recognition. Six listeners using the Med-El/CIS-Link implant showed significantly better performance for monosyllabic words and consonant recognition at higher rates of stimulation (2,100 pps/ch) compared to lower rates (< 800 pps/ch). In one out of a series of four experiments by Nie et al. (2006), stimulus rates were varied from 1,000 pps/ch, 2,000 pps/ch and 4,000 pps/ch, and speech recognition outcomes were obtained from five subjects using the MedEl Combi 40+ CI. Trends of improvement were seen in consonant, vowel and sentence recognition tests in quiet, but not in sentence recognition tests in noise. A study by Buechner et al. (2009) also observed an advantage using high rates of stimulation (1,666 pps/ch) using an n-of-m strategy resulting in significant improvements over rates of 833 pps/ch when subjects used either the CIS strategy or the n-of-m strategy for 20 Clarion implant users.
However, higher stimulation rates have not resulted in improved speech perception performance in all studies [1,5,11,12]. Many other studies have shown limited or no benefits for higher rates of stimulation possibly caused by increased interactions between electrodes and minor effects of additional temporal information with higher rates on perception [9,11]. Friesen et al. (2005) measured phoneme and sentence recognition ability while varying the stimulation rate in 12 listeners using different types of CIs (Clarion C1, Clarion C2 and Nucleus 24). All of the processors were fit with the Continuous Interleaved Sampling (CIS) strategy. Speech recognition performance increased from a rate of 220 pps/ch to 400 pps/ch, but no significant change in speech recognition was seen for stimulation rates that increased from 400 pps/ch to 1,600 pps/ch in the Clarion C1 device. For the Clarion C2 and Nucleus 24 devices, there was no significant improvement in speech perception across any of the stimulation rates. Vandali et al. (2000) investigated the effect of varying stimulation rate from 250, 807, and 1,615 pps/ch on speech comprehension of five listeners using the Nucleus 24 cochlear implant. Open-set monosyllabic words in quiet and open-set sentences at different signal-to-noise ratios were used. In their data, no statistical differences between 250 and 807 pps/ch were observed in any of the speech perception tests, and significantly poorer performance was obtained for the 1,615 pps/ch rate for some tests. Questionnaires from the subjects also revealed preferences for lower rates of stimulation compared to the 1615 pps/ch rate for most conditions. In a more recent study, Shannon et al. (2011) investigated the effect of stimulation rate on speech perception for seven Clarion users in both quiet and in noise conditions. All speech processors were programed with the CIS speech coding strategy. The stimulation rate varied from 600 pps/ch to 4,800 pps/ch and the number of active electrodes varied between 4 and 16. Again, no significant advantage associated with high rates for speech perception was found in speech recognition except for a small improvement in vowel perception in quiet conditions. There was also a small significantly higher subjective preference as stimulation rates increased only from 1,200 pps/ch to 2,400 pps/ch. Arora et al. (2009) focused on low to moderate stimulation rates of 275, 350, 500, and 900 pps/ch and compared speech perception performance for eight subjects with the Nucleus CI24 cochlear implant using the ESPrit 3G processor. Most of the subjects showed a preference for 500 pps/ch and better performance on speech perception in noise with comparatively higher rates of 500 or 900 pps/ch. There was, however, no significant effect of rate was found for monosyllablic word tests.
These studies suggest that no single stimulation rate has been found to be optimal for CI patients. Different conclusions have been made as a result of various factors such as types of devices and signal processing strategies, number of adjustable parameters, speech perception test materials used, and heterogeneity in subjects that considerably affect listeners’ overall performance on speech perception tests. These equivocal findings and the limitations of controlling such variables in these kinds of studies leave the question of what is an optimal stimulation rate still unanswered.
The object of the present study was to investigate various combinations of stimulation rates and the number of maxima within the n-of-m speech processing strategy in the Nucleus 24 cochlear implant system. Practical combinations of stimulation rate and number of maxima using a simulation technique were presented to listeners with normal hearing to determine their performance on a consonant and vowel perception test. By analyzing confusion matrices, we ascertained which minimal pairs were subject to the most confusion and what types of error patterns could be identified in an attempt to get closer to identifying an ideal combination of stimulation rate and number of maxima for improved speech perception. The specific research objectives for this study were to:
Determine which combination(s) of stimulation rate and number of maxima parameters result in improved consonant and vowel perception,
Determine the hierarchy of confusability among minimal pairs, and
Investigate the percentage of distinctive feature information that is transmitted through a simulated CI system.
METHODS
Participants
A power analysis using the G*power program (33) revealed a total sample of 18 participants would be required to have a power level of 0.95. Eighteen young adults (4 males and 14 females) ranging in age from 24 to 49 years (M =26.3, SD =5.94) participated in this study. All participants were native speakers of American English and had negative history of neurological or cognitive defects. A pure-tone air-conduction screening at 20 dB HL at the octave frequencies from 500 Hz through 4 kHz bilaterally proved that individuals had hearing within normal limits, and tympanometry indicated normal middle ear function as evidenced by a normal tympanogram (Type A) bilaterally.
CI simulation
The application “Cochlear Implant Simulation” version 2.0 (34) developed at the University of Granada in Spain was utilized to produce the simulated stimuli. Cochlear Implant Simulation is a software application that simulates sounds through a CI using a computer with a Windows operating system. An attempt was made to create stimuli that were similar to those processed by the ACE strategy in the Nucleus cochlear implant system. The following parameters in the simulation software were adjusted to create the stimuli. Input frequency range, defined as fMin and fMax, processed by the simulation system was set from 150 Hz to 8 kHz. Incoming spectral ranges were separated into the 22 bandpass filters which were composed of the same bandwidths in the logarithmic scale of frequency. This resulted in narrower filter bandwidths at lower frequency ranges and broader filter bandwidths at higher frequency ranges. For example, the center frequency and bandwidth were at 171 Hz and 42 Hz for the lowest frequency band and at 7,668 Hz and 672 Hz for the highest frequency band, respectively. Although the commercial ACE strategy uses Fast Fourier Transform (FFT) based filter bank analysis, an Infinite Impulse Response (IIR) filter was used with envelope detection based on rectification and low-pass filtering (Rect-LP+IIR), taking into account the finding of no critical differences in speech perception between two filter sets in certain conditions [35]. In this system, the functional effect of the stimulation rate is simulated by resampling the speech envelopes with the same sampling frequency as the desired stimulation rate [34]. With the assumption that the slim straight array was used, the cochlear implant length parameter had a value of 20 mm, and the number of inserted electrodes was 22. As the purpose of the study was to focus on the influence of the number of maxima and stimulation rate, other unrelated parameters such as channel interaction and synchronization were not manipulated.
In the present study, 12 simulated stimulus sets of consonants and vowels were created by varying the stimulation rates and the number of maxima using the parameters described above. Table 1 shows four different rates (500, 900, 1,200, and 1,800) were used, each employing three different sets of maxima in accordance with the acceptable parameters of the ACE strategy. All of these combinations of parameters are adjustable in the commercial mapping software (Custom Sound 4.3) provided by Cochlear Americas.
Stimuli
Vowel stimuli were selected from materials recorded by Hillenbrand et al. (1995). Stimuli spoken by 4 speakers (2 male and 2 female talkers) each of 12 medial vowels (i, ɪ, ɛ, æ, u, ʊ, ɑ, ʌ, ɔ, ɝ˛ o, e) in an /hVd/ context were randomly presented in a 12 token-closed-set (heed, hid, head, had, who’d, hood, hod, hud, hawed, heard, hoed, hayed). Each block of vowel test material was composed of 48 tokens (4 speakers×12 vowels), and a total of 12 blocks (4 rates×3 maxima) was measured for each subject.
For the consonant recognition test, 20 medial consonant stimuli (b, d, g, p, t, k, m, n, l, r, f, v, s, z, ∫, ʧ, ð, ʤ, w, j) recorded by Shannon et al. (1999) were used. The stimuli produced by 4 speakers (2 male and 2 female) in an /aCa/ format (aba, ada, aga, apa, ata, aka, ama, ana, ala, ara, afa, ava, asa, aza, asha, acha, atha, aja, awa, aya) were randomly presented. Each block of stimuli consisted of 80 tokens (4 speakers×20 consonants), and 12 blocks (4 rates×3 maxima) were implemented for each subject.
Procedure
All subjects signed an informed consent approved by the Institutional Review Board from The University of Memphis. The consonant and vowel recognition tests were presented in a double-walled sound treated booth meeting ANSI standard (S3.1-1999) in the Speech Perception Assessment Laboratory at the University of Memphis. Subjects were seated in the sound booth with access to a computer monitor and a mouse. A Graphic User Interface (GUI) was developed and controlled by MATLAB® 2013 (The MathWorks, Inc., Natick, MA) for the consonant and vowel tests so that the subjects’ responses were stored automatically in the system. The stimuli were routed from a laptop computer outside the booth through a GSI-61 audiometer to the loudspeaker 1m away from the listener. For implementation of the phoneme tests, 12 test blocks were presented in random order across the subjects to avoid an order effect, and the stimuli within the vowel and consonant tests were also presented in random sequence. For these assignments, random functions were utilized with Microsoft Excel.
Each subject listened to 12 lists of consonants and vowels each presented with a specific combination of stimulation rate and number of maxima. A practice session preceded the main tests using unprocessed stimuli to make sure subjects were familiar with the procedure. The consonant and vowel tests were administered in an alternative forced choice (AFC) procedure (vowels: 12 AFC, consonants: 20 AFC). The subjects were asked to click on the item on the computer screen that matched the stimulus heard from the talker. The stimuli were presented at the Most Comfortable Level (MCL) for each individual, and subjects were encouraged to guess when making decisions without time limits. Participants were not given feedback on their responses.
Data analysis
Mean percent correct scores for the recognition of each phoneme in all conditions were calculated to determine which phonemes were easily or poorly identified. Additionally, a hierarchy of confusability between the target phonemes and responses was determined. Pairs of target stimuli and subjects’ corresponding responses were arranged in order of inaccuracy. In addition, group mean percent correct scores as a function of different stimulus conditions were calculated by combining all participants’ results into a single confusion matrix to determine which combinations of stimulation rate and number of maxima parameters yielded improved consonant and vowel perception.
Results were further analyzed to determine the amount of distinctive feature information that contributed to specific segments of phoneme perception. Information Transfer (IT) analysis, based on Shannon’s development of information theory [36], categorizes confusion matrices according to distinctive features and computes the ratio between the number of bits detected by the listener and the number of bits available in the stimuli. Through this process, we determined the proportion of the distinctive features that was received by the listeners. For consonant classification, the three features of voicing, place of articulation, and manner of articulation were applied. Table 2 shows the classification for consonants in terms of these three distinctive features. For classification of vowels, the four features of height, backness, r-coloring and tense were employed (Table 3). In order to run the IT analysis for consonants or vowels, all 18 subjects’ confusion matrices were pooled by the 12 conditions and combined to form a single confusion matrix. This single response-to-stimulus matrix was analyzed using the Feature Information Xfer (FIX) program developed at University College London. For most of the statistical comparisons, Analyses of Variance (ANOVAs) were conducted using an a priori significance level of p <0.05 using IBM SPSS (v.23).
RESULTS
Optimal combinations of stimulation rate and number of maxima
Figure 1 displays the listeners’ identification performance for the consonant and vowel stimuli. Regardless of parameter combination presented, listeners’ had higher accuracy of identification of consonants compared to vowels with group mean scores for all consonants (M =73.211, SD =6.299) significantly higher than that of all vowels (M =47.578, SD =11.755), t(215) =−27.652, p <0.001. For the consonant test, a repeated measures ANOVA revealed a significant main effect for the 12 different stimuli [F(11, 187) =2.928, p <0.001]. Bonferroni pairwise comparisons revealed that only scores for the 1,800/6 condition (rate =1,800 pps; number of maxima =6) were higher than those for the 500/20 condition (p =0.043). There was also a significant main effect for the vowel stimuli [F(11, 187)=2.255, p =0.013] for one condition. Bonferroni pairwise comparisons for the vowel test revealed that only scores for the 900/8 condition were significantly higher than those for 500/20 (p =0.006).

Average of all 18 subjects’ percent correct scores for consonant (dark grey) and vowel (light grey) recognition as a function of CI mapping configuration. The combinations of stimulation rate and the number of maxima are represented in order on the X-axis.
Additional repeated measure ANOVAs were conducted to look at the independent effect of stimulation rate on vowel and consonant perception. Phoneme perception scores for 4 of the 12 stimulus conditions that have a maxima of 8, but vary with stimulation rates (500/8, 900/8, 1,200/8, 1,800/8), were compared. The results showed that a significant main effect of stimulation rate was not found for consonant [F(3, 42)=0.055, p =0.983] or vowel [F(3, 42)=1.453, p =0.241] perception.
Confusion patterns of CI simulated speech
To investigate confusability among phonemes, first all listeners’ confusion matrixes were pooled together, and one grouped confusion matrix was constructed to calculate percentage of scores for individual consonants and vowels (Figure 2 and 3, respectively). In the matrixes, the phonemes presented are represented along the y-axis while responses from the listeners are represented along the x-axis. Correct responses lie along the diagonal of the confusion matrixes. Correct scores for individual phonemes were then computed in percentage by dividing the number of correct responses by the total number of presentations for each phoneme.

Confusion matrix for consonants. The rows represent the presented consonants in an /aCa/ context along with the corresponding International Phonetic Alphabet (IPA) symbols. The columns represent the listeners’ counted responses. Correct responses lie along the diagonal of the confusion matrix. The total number of presentations and responses are indicated at the end of the matrix.
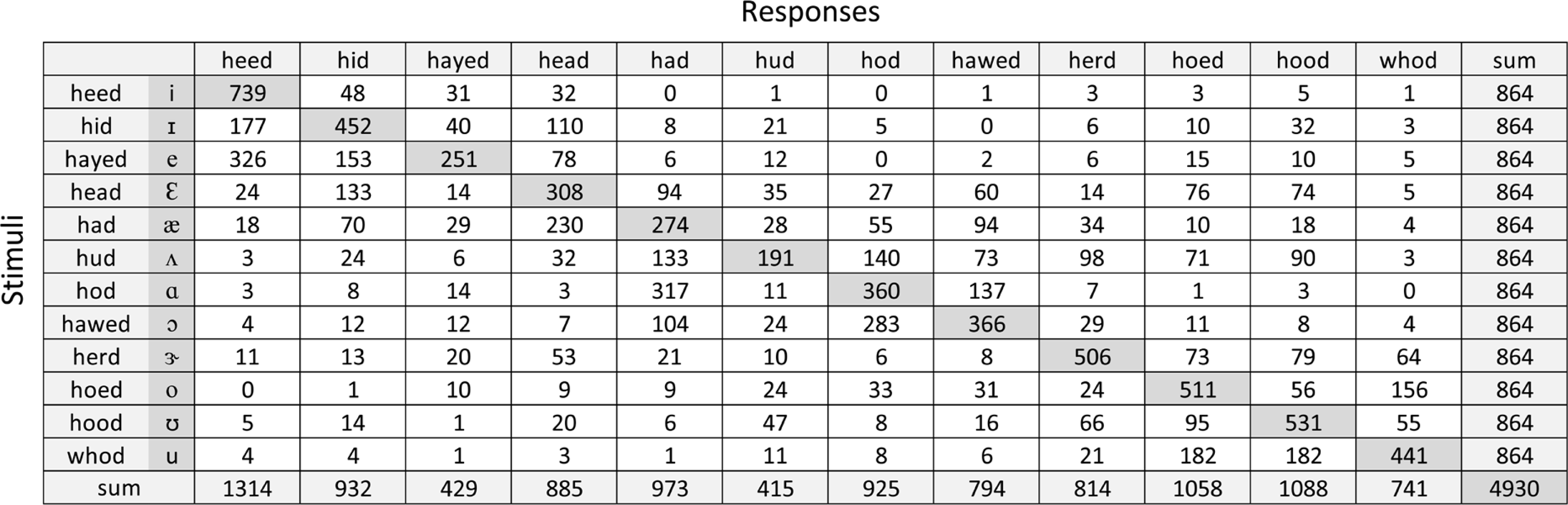
Confusion matrix for vowels. The rows represent the presented consonants in a /hVd/ context along with the corresponding IPA symbols. The columns represent the listeners’ counted responses. Correct responses lie along the diagonal of the confusion matrix. The total number of presentations and responses are indicated at the end of the matrix.
Percent correct scores for individual consonants are shown in Figure 4. Identification scores varied depending on the specific consonants presented. For example, the phonemes/ð/, /l/ and /r/ were poorly identified (<40%), while /t/, /g/, /p/, /z/, /f/, /v/ and /y/ were identified with much greater accuracy (>90%). The consonant confusion matrix (see in Figure 2) revealed that /l/ and /r/ were often misperceived as /v/ or /w/, while /ð/ tended to be misperceived as /v/. Asymmetric confusion patterns were typically observed in our data set which is consistent with those reported in classic studies by [37,38]. For instance, in this study /d/ was frequently confused with /g/ (84% total error rate), yet misidentification of /g/ for /d/ had only a 16.3% total error rate. This indicates significant response biases of listeners when they faced ambiguous and confusable phonological cues [39]. Overall, the consonant /v/ was the predominant response (12.91% of the total responses) used by our subjects.
Figure 5 shows listeners’ vowel identification was relatively poor when identifying /ʌ/, /e/, /æ/, and /ɛ/ (<40%), but better performance was observed for correctly identifying /i/ (>80%). In general, the overall vowel confusion patterns were related to place of articulation suggesting that vowels were more likely to be confused with another vowel in the same category. For example, listeners often responded with the frontal vowel /i/ when the frontal /e/ and /ɪ/ were presented. Likewise, the vowel /ɛ/ was more likely confused with /ɪ/ and /æ/; /ʌ/ was more likely confused with either /ɑ/ or /æ/; and /u/ was most often confused with /ʊ/ or /o/ (see in Figure 3).
Information transfer (IT)
Consonants
To determine the proportion of the features contributing to phoneme identification, individual listener’s ITs were calculated. Consonant ITs are shown in Figure 6 as a function of the 12 different stimuli. A two-way repeated measures ANOVA was conducted with the 12 stimuli and three consonant features (voicing, manner, and place) as the two within-subject variables and IT as the dependent variable. Significant main effects were found for the stimuli [F(11, 187)=3.424, p <0.001] and features [F(2, 34)=164.894, p <0.001] with a significant interaction effect between the two [F(22, 374)=5.801, p <0.001]. Adjustment for multiple comparisons with a Bonferroni post hoc analysis revealed the amount of IT for the 1,200/10 parameter combination was higher than for 500/20 (p <0.05). In addition, the place feature (55.34%) was significantly weaker than either voicing (79.89%) or manner (77.76%) features in terms of the amount of information transmitted (p <0.05).

Grand mean percentage of information transmitted for consonant features (voicing, manner, and place) as a function of CI mapping configurations. The combination of stimulation rates and the number of maxima are represented in order on the X-axis.
The specific classification of the consonant feature characteristics of manner (stop, fricative, affricate, nasal, and glide) and place (labial, alveolar, palatal, and velar) were further analyzed to investigate the amount of information transmitted with CI vocoded speech. To this end, all ITs across the 12 stimuli were pooled together based on the classification of manner and place of articulation. Figure 7A shows that for the manner feature, stops were transmitted best followed by affricates, fricatives, nasals, and glides. A one-way ANOVA revealed a significant difference in IT for the five different manner features (stop, fricative, affricate, nasal, and glide) [F(4, 1,075)=146.379, p <0.001]. Post hoc Tukey HSD revealed that all-pairwise comparisons of manner features were significantly different from each other (p <0.05), except for the nasal and glide pair (p =0.902). ITs for the place feature were also significantly different from each other [F(3,860) =56.199, p <0.001]. Figure 7B shows IT for velars was best transmitted followed by palatals, labials, and alveolars. A Tukey HSD post-hoc comparison with an alpha level of 0.05 revealed that IT for labials was significantly higher than for alveolars and velars, but not for palatals. Alveolars were significantly weaker than any other place features. There was no statistical difference in IT between palatals and velars.
Vowels
IT for the four vowel classifications across the 12 stimulus are represented in Figure 8. A repeated measures ANOVA indicated a significant main effect of stimuli [F(11,187) =2.802, p =0.002] and features [F(3, 51)=16.654, p <0.001] along with an interaction effect between the two [F(33,561) =3.152, p <0.001]. Bonferroni pairwise comparisons revealed that IT for the 1,800/4 and 1,800/8 parameter combinations were significantly higher than for the 500/14 combination (p <0.05). For comparison of features of vowels, backness (44.35%) was transmitted best followed by r-coloring (38.59%), height (35.76%), and tense (23.6%), respectively. IT for backness was significantly higher than either height or tense, but not for r-coloring in the pairwise comparison using the Bonferroni adjustment (p <0.05).
DISCUSSION
This study was designed to investigate optimal combinations of stimulation rate and number of maxima using CI processed speech. Our findings showed that only a few simulated parameter combinations resulted in significantly higher phoneme identification than others. Corresponding error patterns were also determined by creating confusion matrixes and estimating the amount of information transferred.
Optimal combinations of stimulation rate and number of maxima
Although differences in overall identification scores across the 12 stimulus conditions were not remarkable, a few parameter combinations clearly revealed significantly better performance (1,800/6 >500/20 for consonant identification, 900/8>500/20 for vowel perception). These findings showed comparatively fewer number of maxima (6 and 8) resulted in superior outcomes over a higher number of maxima [20] when they were coupled with relatively high stimulation rates (1,800 pps and 900 pps>500 pps). This interaction between number of maxima and stimulation rate agrees with the trade-off relationship seen between spectral and temporal information that has been found in previous CI studies [9,40–42]. Such studies have shown trends in temporal-spectral trade-offs in speech recognition when systematically co-varying spectral and temporal information. Even though this consistent trend was not observed for all stimuli in our results, the temporal-spectral trade-off was observed assuming that higher stimulation rates might compensate for a reduced number of maxima in speech recognition.
Despite the theoretical advantages that an increase in the number of maxima and higher stimulation rates would have for greater accessibility of spectral and temporal cues, the optimal parameter combinations found here, however, were not ones that occurred at the extreme ends of the range in parameter sets. For example, phoneme identification scores for the 1,800/8 condition were not significantly higher than those for the 1,800/6 condition. In addition, the optimal parameter combinations differed based on the type of stimulus used (1,800/6 for consonants, 900/8 for vowels) suggesting that different parameter combinations are needed for improved consonant perception compared to those that result in better vowel perception. Thus, it is possible that only using one parameter combination for each map could have a potential detrimental effect on speech perception by providing an unnecessarily large amount of spectral or temporal cues. Furthermore, due to the complexity of parameter interactions, CI listeners’ optimal maps may not be simply found at the extreme end of given parameter set, but rather they might be more appropriate if a range of parameter combinations is provided that plausibly improves speech perception for different types of stimuli. This is to some extent consistent with the recommended defaults from CI manufacturers. For example, Cochlear Americas typically recommends the parameter combination of 900/8 for the ACE strategy, but they also provide the option for a wide range of additional parameter combinations in their clinical guide book [43].
Speech recognition requires both spectral and temporal resolution. The contribution of both of these parameters in recognizing consonants and vowels is not equal due to the different characteristics of phonetic landmarks in consonants and vowels. While consonant recognition predominantly depends on temporal cues, vowel recognition depends more on spectral cues available in speech [40,41]. Our findings agree with this notion for consonants (1,800/6>500/20), but not for vowels (900/8>500/20). This disagreement in vowel perception may stem from the interaction effect between the two parameters. It is also reasonable to suppose that 8 maxima may be sufficient enough to provide spectral information, without needing an unnecessarily high number of maxima [20]. Similar findings were observed by Dorman et al. (2002) who found that m maximum vowel recognition was reached with as few as 3 maxima using CI simulation. These findings in combination support the recommendation by Cochlear Americas to use a stimulation rate of 900 pps and 8 maxima as the default setting.
Confusion patterns of CI speech
The consonants /ð/, /l/, and /r/ were perceived with lower accuracy than the other consonants tested in this study. Previous phoneme recognition studies have reported that listeners typically have a large number of errors when perceiving /ð/ [21,37,38] even under well-controlled conditions using un-processed stimuli in listeners with normal hearing [44]. The poorly perceived liquids, /l/ and /r/, tend to elicit responses of glides such as /w/. The /l/ and /r/ are produced with an obstructed air stream, but not as much as is seen in the articulation of stop consonants. The major perceptual cues for those semivowels are formant structures. Thus, it could be assumed that degraded formant structures of those vowel-like consonants by CI processing caused the listeners to confuse them with another semivowel. Regardless of the type of consonants presented, many of our listeners responded with /v/, especially when the stimulus was /ð/ or the sonorants (/r/, /l/, /m/ and /n/). This implies that the degraded acoustic properties of these CI processed consonants (characteristics of fricative noise in /ð/, and low of frequency of voicing in sonorants) are similar to those of /v/.
For vowel confusions, listeners had poor perception of the vowels /ʌ/, /e/, /æ/, and /ɛ/ (<40%) and tended to show confusion patterns that were associated with height features in place of articulation. As mentioned previously, the vowel /ɛ/ was more likely confused with /ɪ/ and /æ/; the vowel /ʌ/ was confused most with either /ɑ/or/æ/; and the vowel /u/ was confused with /ʊ/or/o/. It is likely that these errors occurred because listeners lacked the perception of the height feature. The inability to perceive height was also seen in our IT analysis that determines the amount of bits transferred across distinctive features described below.
Information transfer (IT)
The amount of IT for 1,200/10 was higher than that for 500/20 in consonants, and IT for 1,800/4 or 1,800/8 was higher than that for 500/14 for vowels. These results differ from that of our percent correct comparisons where performance for 1,800/6 was better than 500/20 for consonants, and 900/8 was greater than 500/20 in vowels. This stems from the underlying rationale of the two analyses employed. Percent correct measurements account for the scores correctly identified, where one can obtain credit if his/her response is correct, even if some of the features in the phoneme were missed. In comparison, IT computes the bits of distinctive features transferred in the phoneme. Thus, unequal units computed between the two estimates resulted in discrepancies. The variation in the results of the two analyses may also potentially be caused by the significant interaction effect between the distinctive features and the 12 stimuli. Unlike percent correct comparisons which have only one dependent variable of the score, a number of features (e.g., voicing, manner and place) were involved in the IT estimations, each of which might have resulted in a different effect on the information transferred. Thus, interaction effects from the multiple variables in the IT analysis might have contributed to the different results compared to those of the percent correct score
Among the three consonant articulatory features, the transmission of manner and voicing is more dependent on temporal resolution, while place relies more heavily on spectral information [40,41,45]. Our findings agree with previous studies [21,40,46–49] that found less information transfer for the place feature than the other two consonant features (manner and voicing). It has been noted that this pattern is primarily attributed to the limited number of electrodes in a CI signal processing strategy for frequency matching [45]. Given that this pattern of IT has also been seen in several other studies that did not use CIs [37,39,50], it is more likely due to the fact that the representation of the acoustic nature of place cues is relatively weaker than other articulatory features, resulting in limited acoustic information about place cues. In fact, the acoustic correlate of place of articulation is susceptible to the corresponding manner of articulation [45]. In this regard, Munson et al. (2003) stated that the estimate of IT for the place feature provides limited information on specific acoustic parameters.
Phonological vowel features are considered to be closely related to phonetic formants; height, backness, and r-coloring are reflected with F1, F2, and F3 respectively. We found that listeners were better able to access backness information than height information, suggesting that CI listeners may be in need of additional F1 cues to improve vowel identification. This finding is in agreement with previous studies [21,46,51]. Munson et al. (2003) argued that speech processing strategies designed to better represent F1 cues would be beneficial for CI users who show poor performance. For example, as pitch discrimination is heavily dependent on activation of distinct electrodes, allocating more channels in the low frequencies could be a plausible option that enhances the F1 cue [51]. However, caution should be exerted when drawing conclusions about the lack of F1 cues for CI users’ speech perception because this type of vowel IT pattern has also been found in studies that did not use CI signal processing [50].
Clinical implications, limitations, and directions for future research
In this study we examined 12 parameter combinations of number of maxima and stimulation rate as well as corresponding error patterns uses CI simulation in listeners with normal hearing. The goal was to identify an optimal parameter setting that would result in improved speech perception. Although our speech recognition outcomes associated with parameter variation were not strong for many of the combinations, some of them (e.g., 900/8, 1,800/6) were clearly better than others (e.g., 500/20). Considering individual variability among patients and time constraints in clinics, providing CI patients with parameter sets that maximize speech intelligibility is not an easy process. The findings reported here should help audiologists, especially those who work in busy clinics, improve CI mapping for their patients. The optimal parameter sets we found could be potentially good options to try prior to other maps. In addition, the error patterns obtained here for vocoded speech may contribute to not only a better understanding of CI patients’ perceptual capabilities, but also of refinements in developing speech coding algorithms for improving intelligibility.
There are, however, several limitations that are worth noting in the current study. First, due to the difference in mechanisms between electrical stimulation in CI users and acoustic simulation in listeners with normal hearing, discrepancies in the two approaches are expected. It is necessary to extend this investigation to actual CI patients to improve the external validity of our findings. Secondly, optimal parameter settings vary from person to person [1,10]. Thus, it would be worth analyzing and providing insight into CI users’ optimal parameter settings and error patterns on an individual basis. Lastly, using the context of /aCa/ and /hVd/ potentially limited the phoneme recognition results as has been documented in previous studies [38,52]. In fact, the /iCi/ context has been found to be more sensitive to variation of stimulation rate than /aCa/[8]. In addition, different optimal parameter combinations might have been found if other configurations of maxima and stimulation rate or other mapping parameters were included. However, the addition of such variables would likely cause complications and interaction effects. Further studies on different large scale assessments are needed.
Notes
The author has no conflict of interests.
Acknowledgements
The authors thank Dr. de la Torre Vega for providing the CI simulation tool with materials and Dr. Shannon for providing the stimulus set.