Attention-Controlled Working Memory Measures to Assess Listening Effort
Article information
Abstract
Purpose
To assess working memory (WM) performance using novel tasks that included controlled-attention functions of updating of information, selective attention, task monitoring, and multiple task coordination as well as relating performance to perceived listening effort.
Methods
Sixteen adults with normal hearing participated in two tasks:1) the attention switching (AS) task that required participants to categorize digits rapidly and recall totals of each category and 2) the working memory span task (WMST) that involved storing and recalling digits while performing a secondary sentence verification task. Each experiment was conducted in quiet and in multi-talker babble.
Results
In the AS task, performance was worse when noise was added and when digit categories changed. In the WMST, digit recall accuracy was worse in noise, but reaction times (RTs) were not affected.
Conclusions
The results of this experiment show that accuracy and RT performance on auditory cognitive tasks generally worsens in background noise, even when intelligibility remains high. This suggests that background noise increases WM load and the decline in performance can be interpreted as an increase in listening effort. The specific integration of attentional control in these tasks, especially the AS task, may 1) have increased the sensitivity over traditional WM tasks and 2) allow for the ability to differentiate between peripheral and central mechanisms.
INTRODUCTION
Interfering sounds pose challenges to successful verbal interactions, especially if the listener has a hearing loss. For several decades, quantifying speech intelligibility in noise has been the preferred method to assess listener performance [1]. Several methods have been used, including the Bamford-Kowal-Bench Speech-in-Noise Test [2,3], the Hearing in Noise Test [4], and the Quick Speech-in-Noise Test [5]. Typically, the signal-to-noise ratio (SNR) at which a listener repeats 50% of the words in a sentence correctly is measured to evaluate performance. The major drawback of this category of speech testing, however, is that it primarily measures the listener’s ability to briefly remember and repeat the sentences or words; such tests are not designed to measure the listener’s speech comprehension, processing load, and/or listening effort that are required in real-world listening situations. Moreover, the SNRs that result in 50% performance on these tasks can be up to 25 dB lower than typical everyday listening, contributing to the low ecological value of traditional speech-in-noise testing [6].
In a real-world listening environment, it is uncommon to encounter a situation in which a person is required to repeat back a sequence of words or sentences with no additional processing involved; virtually all verbal interactions require some level of language comprehension. Therefore, listening tests that engage higher levels of processing may provide a more realistic method to measure a person’s listening abilities. Since listening comprehension involves simultaneous bottom-up and top-down processing, it is generally believed that higher order cognitive systems, including attention and memory, are involved in these automatic and controlled processes [7]. Of particular importance, therefore, is the construct of working memory when evaluating the resources required for complex listening situations that are necessary for everyday listening environments.
Working memory (WM) describes a mechanism used to store and process auditory, verbal and linguistic information [8]. The concept of WM has evolved to describe the cognitive systems where a limited amount of information can be temporarily stored and processed to achieve some mental activity. The WM system is thought to be a crucial aspect of comprehension; a listener first needs to process the speech signal and then maintain the intermediate products of speech processing until the complete message is understood [9]. In this sense, speech intelligibility can be considered a necessary precursor to comprehension. Accordingly, it has been of interest to study the interaction between working memory and listening comprehension [10,11].
Supporting this notion, it has been shown that while cognitive factors have a role in speech intelligibility in noise, the extent of their contribution has been mixed across studies [12–14]. Moreover, the measurement of listeners’ intelligibility in quiet may not be sensitive to cognitive factors impacting comprehension [15–17] suggesting that cognitive factors are relied upon more heavily as the listening condition becomes more difficult. Intelligibility focuses on word-and/or sentence-level recognition, often measured by rote recall and is highly impacted by bottom-up factors (e.g., acoustics). Comprehension, on the other hand, focuses on understanding the intended meaning of discourse and is therefore influenced by both bottom-up and top-down processes [18].
Baddeley et al. employed a verbal sentence recall task with concurrent WM tasks, thus involving executive (e.g., coordination, allocation, focus of attention) processes [15]. They found that the processes engaged in sentence recall (i.e., speech intelligibility tests) occur automatically, with minimal involvement of executive processes. The influence of cognitive factors and hearing loss have only been investigated recently [19]. This may be in part because previous research in hearing science has emphasized predicting speech intelligibility using simple, controlled stimuli (such as pure tones, consonant-vowel syllables, nonsense syllables, words, and simple sentences) in order to understand the acoustic-phonetic cues of the speech and their influence on speech perception.
Rönnberg proposed the Ease of Language Understanding (ELU) model to account for the role of WM in language processing [20]. The ELU model has been used successfully to account for the effects of hearing impairment and/or listening condition on cognitive load during speech comprehension [17,21,22]. According to the ELU model, incoming linguistic information is multimodal (e.g., phonology, semantics, syntax, visual, etc.) and is quickly bound together by an episodic buffer (called Rapid Automatic Multimodal Binding of Phonology, or RAMBPHO). Phonological information extracted at the RAMBPHO stage is assumed to be compared with phonological representation in long-term memory (LTM). If the phonological information is precise, then speech comprehension is achieved with implicit (automatic) processing by matching the input with stored phonological representations in the LTM. However, when there is distortion or missing information in the phonological representation, explicit processing is required to understand speech. Explicit processing is similar to top-down processing in that additional semantic or contextual information is retrieved from LTM to replace the missing phonological information. Cognitive resources for attention that control maintenance and processing in WM are used during explicit processing. It is generally believed that the mismatch between phonological input and representation in LTM occurs commonly in hearing impaired listeners or during adverse listening conditions [23]. When a mismatch occurs, explicit processing plays a crucial role in speech comprehension. Explicit processing of speech comprehension is thought to be slow and effortful, involving WM and attention to a much greater degree. The role of WM is thought to play an important part in an individual’s ability to mitigate the effects of a phonological/semantic mismatch. Those with high working memory capacity (WMC) are better able to inhibit the mismatched representation in their semantic memory [17]. In complex listening situations where one must attend to multiple speech inputs, listeners must coordinate both bottom-up and top-down mechanisms, including perceptual processing, WM, and attention to understand speech [21]. Rönnberg et al. suggested that individuals with high WMC are more efficient listeners in complex listening situations [17]. For example, in a task that requires listening to a target speaker among multiple talkers, those with high WMC are able to utilize more cognitive resources for both phonological and semantic processing requirements of the task. This allows them to better inhibit the competing talkers while focusing on the target speaker, which in turn facilitates a deeper understanding of the message and helps create more durable episodic memory traces [17].
Allocation of attentional resources plays a key role in WM processes. Several models of WM incorporate a central processing unit that encumbers attentional resources in order to carry out executive functions [24–30]. Most WM models suggest that control of attention is crucial for active rehearsal of stored items and processing of information in complex WM tasks [27,31]. Moreover, it has been related to an ability to maintain more items in WM while performing other cognitive tasks [32]. The more efficient the listener is in switching his/her focus of attention and updating the items in active WM, the more likely an increased number of items will be recalled. Barrouillet, Bernardin and Camos proposed a Time-Based Resource-Sharing model whereby attention is switched rapidly between processing and storage during a task to maintain memory traces [33]. In this model, a central bottleneck of attention was proposed as a limiting factor in WM performance. Other models have also described the role of attention as being quite dynamic; it must be able to be focused, divided, switched between tasks, “zoomed in” or “zoomed out,” and used to activate LTM stores that can be linked with short-term stores for processing [26,29,33–35]. Collectively, these models share the common theme that attentional control is a vital aspect of WM and the ability to allocate attentional resources efficiently is a major factor in dictating WM performance.
In recent years, an increasing understanding of the modality-specific aspects of WM has been articulated. The idea of central storage has been maintained largely for categorical information. Added to this, however, is the notion of a peripheral store that holds certain types of modality-specific information. Given that sensory information decays quickly, the categorical information from central storage is required for recall. Thus, WMC is the aggregate capacities of these two stores. Attention, however, is a resource available to the central store only [36]. As such, modality-specific tasks carefully requiring differing levels of attention can deduce performance in a WM task attributed to the central and peripheral stores, allowing a better understanding of each mechanism. Under this assumption, tasks that rely heavily upon attentional processes can considered to be a more direct assessment of the central storage mechanisms.
The difficulty of the task and/or the environment in which the information is presented, as well as the capacity inherent to the individual, modulates the amount of resources an individual must encumber; more difficult scenarios require the individual to utilize more resources (i.e., increase effort) to perform the task [17,21]. Once these resources have been recruited to overcome distracting inputs in the environment via explicit processing, fewer resources remain in WM to actually perform said task. Simultaneously increasing resource allocation while having fewer resources to draw upon ultimately affects the accuracy and speed of speech information processing. Hence, listening effort can directly reflect the magnitude of cognitive resources utilized to understand speech. Therefore, if the goal is to quantify the cognitive effort expended in a listening situation, it is reasonable to use cognitive measures, particularly those related to WM and attention. It has been shown that if the processing and storage demands of a given task exceed the available WM resources (i.e., beyond an individual’s WMC), errors such as reduced accuracy, loss of information from temporary storage, and/or slower processing increasingly occurs [15,21].
Several researchers have used a dual-task paradigm to measure listening effort along with speech recognition in noise. Dual-task paradigms are designed under the assumption that performing two concurrent tasks, generally of different modalities, will compete for common cognitive resources. A common protocol for dual-task procedures is speech recognition (primary task) followed by a memory or visual tracking task [12,37–40]. Results can be summarized by the finding that as the primary task increases in difficulty (e.g., adding background noise to speech recognition), performance in the secondary task decreases (e.g., fewer words recalled, reduction in visual tracking accuracy). These results can be interpreted as an increase in listening effort due to a portion of the shared cognitive resources needing to be allotted to the secondary task, thus leaving fewer resources to complete the primary task.
Dual-task experiments have demonstrated the dynamic relationship between cognitive load and speech understanding in noise. While dual-tasks have shown to be effective as objective measurements of effort using different modalities, this experiment was designed to assess the attentional demands placed on auditory WM when listening in an adverse environment. Accordingly, measuring WM and attentional processes can provide valuable information about listening effort. Considering recent evidence demonstrating the modality-specific mechanisms of WM, the tasks developed for this experiment allowed investigation of the auditory modality without interactions from others (e.g., visual). As such, performance on these tasks would directly assess the auditory domain. Tasks designed such that all stimuli are restricted to the auditory domain and include specific attentional control requirements may better inform the relative roles between the peripheral store and the central components of working memory and attention [36].
The aim of this study was to further examine the role that cognitive load plays in regards to listening effort using WM- and attention-related tasks. By collecting objective data on how listeners’ WM processes and attention are affected by background noise, it is possible to develop an understanding of how the presence of noise impacts speech understanding at a cognitive level. A recent study by Lunner et al. showed that when hearing-impaired participants performed a word identification and retrieval task in the presence of low levels of background noise (i.e., 95% identification), performance on the task was significantly improved when digital noise reduction algorithms were implemented [6]. This suggests that even when speech recognition virtually is at ceiling performance, low levels of background noise can hinder performance on other aspects of the tasks where storage and processing occur. Similarly, Rudner reported on a series of experiments that examined the notion of cognitive spare capacity, i.e., the ability to engage in and process spoken information [41]. Using a series of tasks that required participants to process speech stimuli and hold certain components in memory for later recall with varying levels of processing and background noise that did not interfere with intelligibility, the researchers examined how these manipulations affected cognitive spare capacity. The results of these experiments demonstrated that adding low levels of noise reduced cognitive spare capacity, even though intelligibility did not suffer. Additionally, an increased memory load during the tasks proved to reduce cognitive spare capacity as well. These results can be interpreted within the ELU framework [17] suggesting that both extrinsic factors such as noise and intrinsic factors such as increased cognitive demands reduce performance on listening tasks by requiring more effortful, explicit processing to occur. Using objective measures to quantify the cognitive resources required to listen in background noise (i.e., listening effort) would address the high variability of subjective self-reported measures of perceived effort and provide a more robust assessment of the impact of difficult listening environments [42].
To better understand the cognitive mechanisms involved in listening in difficult environments, an auditory-based complex working memory span task (WMST) and an auditory attention switching (AS) task were designed. The WMST designed for this study was a complex auditory WM span task that involved simultaneous processing and storage of auditory information, rather than the traditional dual-task paradigm involving two concurrent tasks of different modalities. This task was adapted from the reading span task [9], which has been widely used in psychology to study WM and found to be highly reliable. Modifications were made to the classic reading span task in order to develop the WMST; the first modification was moving the position of the item to be stored in memory for later recall to the beginning of each sentence. This was done to ensure that storage and processing were occurring simultaneously, compared to the final-word recall condition of the reading span task which requires no storage until the end of the first sentence. The next modification was to change the memory recall item from a word in the sentence to a single numerical digit in order to separate the storage component from the processing component. Using digits rather than words from the sentences removed any contextual clues that may be ascertained from the sentence and creates an entirely new storage bank of information, further dissociating the storage and processing mechanisms required for the task. Similar to the reading span task, participants were required to judge the “truth value” of the sentences as either true or false, rather than simply reading (or hearing) them. The sentences were constructed in a manner that made them either semantically plausible or not. The participants were judging if the sentences were sensible, with “true” sentences being those which were and “false” sentences being those which were not. In order for the participants to be able to determine the truth value of a sentence, they were required to process the entire sentence as a whole. This level of processing served two purposes: 1) the participant needed to demonstrate a clear understanding of speech, and 2) listening to and processing each full sentence limited the rehearsal capabilities of the items stored in memory. Previous researchers have mainly used speech recognition measures to establish the relation between speech recognition and effort, rather than more in-depth speech processing [40,43,44].
In this experiment, the WMST incorporated attentional control by requiring a decision on the truth value of the sentences while retaining unrelated information. Considering the importance of attentional control in complex WM tasks, an auditory attention-switching (AS) task was designed to tax this aspect of WM. The AS task explicitly examined attentional control by measuring a participant’s ability to update and switch between two separate “bins” of information. This task was adapted from Magimairaj and Montgomery [45,46]; similar tasks that require the switching of attention have been widely used in the field of psychology and found to be related to WM and fluid intelligence [31,47]. The AS task in this experiment was designed to measure the listener’s ability to rapidly and accurately switch his or her focus of attention between listening for the items and updating information in WM.
Demands on explicit processing in the WMST and AS tasks were controlled by the independent variables of listening condition (both tasks), stimuli category (AS), and sentence complexity (WMST). To manipulate the difficulty of the listening condition, participants completed the tasks in quiet and in the presence of multi-talker babble (MTB). For the AS task, several hypotheses were made concerning listening condition: 1) recall accuracy at the end of each string of digit presentations would be poorer in the noise condition, which served as the primary task outcome, 2) reaction times (RTs) would be longer in the noise condition, and 3) subjective effort ratings were expected to be higher (more effortful) in the noise condition. The AS task was designed so that the digit presented fell into one of two categories which could either remain the same or switch to the other category for each subsequent presentation. The final hypothesis was that the presentations that switched categories would have longer reaction times (RTs) than those that stayed in the same category.
For the WMST, several main hypotheses were also made concerning noise condition: 1) digit recall accuracy at the end of each block of sentences would be poorer in the noise condition, which served as the primary task outcome, 2) RTs would be longer in the noise condition, and 3) subjective effort ratings would be higher (more effortful) in the noise condition. The WMST was designed to have simple “subject-verb-object” (SVO) and complex “objective-relative” (OR) sentences to measure speech comprehension. This independent variable of sentence complexity led to several further hypotheses: sentence verification accuracy would likely be decreased for the more-difficult OR sentences. Accordingly, it was hypothesized that RTs would be higher for the OR sentences. The addition of MTB however, was not expected to affect sentence verification accuracy since intelligibility for both conditions was intended to be high (SNR=90%).
MATERIALS AND METHODS
Participants
Sixteen adults (mean age, 22.9 years; range: 21 to 29 years) with normal hearing participated in the study. All participants passed hearing screenings for air conduction thresholds at 20 dB HL for octave frequencies from 500–4,000 Hz. Participants also completed an informed consent form and the Six-Item Cognitive Screener [48] before data collection. Persons with hearing loss were excluded from the study. Participants were recruited from the Ohio University student population. This study was approved for use of human subjects by the Ohio University Institutional Review Board.
Stimuli
Digits and MTB were used as stimuli for Experiment 1 (attention-switching task, AS) and Experiment 2 (working memory span task, WMST). Digits one through nine were recorded by a male speaker in a sound attenuating booth using a digital recorder (Marantz PMD661, Mahwah, NJ), a microphone (Sennheiser 845 S, Old Lyme, CT), and digital recording software (Avid Audio Pro Tools 8.1, Burlington, MA). All digits were edited to normalize duration and level using Adobe Audition 3.0 and Pro Tools 8.1. The average duration of all raw recorded digits was 392 ms; this duration was chosen as the target length for each digit and all digits were time compressed or lengthened to make them equal in duration with no audible distortion. All stimuli were normalized to have equal RMS. The MTB was taken from the QuickSIN test [49]. The babble was comprised of 4 talkers (one male, three female), intended to create a realistic simulation of a noisy social environment. In Experiment 2, along with digits and MTB, sentences were also used. Sentences were recorded by the same male speaker as Experiment 1. Each sentence contained 11 words and their durations ranged from 2.18 to 3.39 seconds. Two sentence structures were recorded: a subject-verb object (SVO) and object-relative (OR) structure. “True” and “false” versions of each of these structures were also created (e.g., SVO True: “The girl helped the kitten from the floor onto the pillow” SVO False: “The kitten helped the girl from the floor onto the pillow” OR True: “The kitten that the girl helped onto the pillow was tiny,” and OR False: “The girl that the kitten helped onto the pillow was tiny”). A total of 1,000 sentences were created. The truth value of each sentence was verified by a survey given to native English speakers that asked participants to determine whether a sentence was “true” or “false.” A total of 16 mono-lingual, native English speaking adults completed and returned the survey (none of whom participated in the experiment). They were asked to rate the truth value of each sentence on a scale from 1 to 7 (1=very true, 7=very false). The results of each sentence were tallied. Sentences that received three or more scores in the range of 3–5 were excluded from the study. True or false sentences that received three or more scores claiming they had the opposite truth value were also excluded. These surveys ensured that the sentences presented during the experiments correctly represented the category of true or false in which they were scored.
The digits, MTB, and sentences were calibrated to 60 dB SPL using a Brüel and Kjær (Nærum, Denmark) sound level meter Type 2,250 with Brüel and Kjær half inch, style 4,189, free field microphone, C-weighting network. For calibration, the microphone was placed directly facing the speaker at 0° azimuth with no person or objects positioned around the microphone. The distance from the microphone to the speaker was 3 feet; the height of the speaker and microphone was 3 feet off the ground.
Stimuli presentation
All stimuli were output by a computer soundcard (Creative Labs Soundblaster X-Fi, Milpitas, CA) and routed through a programmable attenuator (Tucker-Davis Technologies PA5, Alachua, FL). The stimuli were then amplified through an audio amplifier (Crown CTs-4200, Elkhart, IN) and presented over a loudspeaker (B&W DM601 S3, West Sussex, UK). Participants were instructed to keep their heads positioned directly in front of the speaker; the placement of the chair in front of the computer facilitated this. All stimuli were presented from a loudspeaker located at 0° azimuth and at a distance of 3 feet from the participant’s head.
Communication between the participant and examiner occurred through an intercom system. Instructions were provided via a touch-screen monitor (3M Microtouch, St. Paul, MN). The subject used a keyboard (Dell, Round Rock, TX) to respond to the stimulus. Responses were recorded through specialized software (Psychology Software Tools E-Prime 2.0 Professional Edition, Sharpsburg, PA).
Experiment 1: Attention switching
In this task, the ability of participants to process and update incoming speech information appropriately was assessed in quiet and in the presence of MTB. Participants were first asked to determine whether a digit belonged in one of two categories, and after an unspecified number of digits had been presented, they were asked to express how many digits were presented for each category. Measuring RT after each presentation and accuracy after each string of digits allowed the assessment of how one can simultaneously categorize and store new information in the presence of background noise. Based on the discussed models of WM, adding low levels of noise to the task increased the cognitive load by drawing some cognitive resources away from the processing task in order to suppress the background noise.
Procedure: SNR assessment
Prior to the AS task, an SNR assessment was employed to determine the level that the MTB interferer must be presented in order to load WM and attention capacities without reducing intelligibility. This assessment found the level of the MTB (or rather, the ratio between the speech and babble) for which ≥ 90% recognition was achieved for digit stimuli; this was done to ensure that each participant performed the task under the same relative load. In this task, the participants were asked to repeat back digits in the presence of MTB starting at 0 dB SNR. Twenty-seven digits were randomly presented in each SNR condition. The level of the digits was kept constant at 60 dB SPL while the level of MTB was adjusted in 2.5 dB steps. The lowest SNR at which each participant was able to achieve ≥ 90% correct recognition was the SNR used for that participant in the noise condition of Experiment 1 (see Table 1 for descriptive statistics).
Attention switching task
The AS task was performed in quiet and in the presence of MTB by all participants; the order was counterbalanced. The MTB level was determined by the participant’s SNR assessment results, as described above. The level of digits was kept constant at 60 dB SPL for both quiet and noise conditions. For any given presentation, the participants were instructed to keep track of the category in which the digit was associated, e.g. “Low” (i.e., 1–4) or “High” (i.e., 6–9). One-hundred milli-seconds after the participant pressed a button, a subsequent digit was presented. Participants were instructed to keep their hand on the designated area marked in front of the keyboard between presses of the space bar; they were told not to leave their hand on or above the space bar. Participants were also instructed to respond as quickly as possible on the task while still maintaining accuracy; there was a 2.5 second maximum RT allowed. The time between the end of the digit presentation and the button press was recorded as the RT. The participant pressed a button to initiate the next trial. This response indicated the participant’s readiness for the next trial. As such, RTs were recorded after each digit trial, but accuracy data were not. Accuracy was tallied at the end of each block when the participant was instructed to recall verbally how many digits were presented from each category (e.g., “5 low, 7 high”). Therefore, accuracy calculations were performed at the string level (i.e., accuracy results were only recorded after an entire string of digits had been presented). The total number of digits in each string ranged randomly from 12–15. See Figure 1 for a representation of the procedural flow.

A schematic representation of the AS task is depicted. A digit, from either a low (1–4) or high category (6–9), was presented at 60 dB SPL through a speaker to participants in quiet and in the presence of MTB. The RT (ms) was a measure of how quickly the participant selected which category (i.e. high or low) a digit belonged to. Following this keystroke, there was a 100 ms gap before the cycle repeated.
The presentations of the digits were categorized as either switch or non-switch; a presentation was labeled as a switch presentation if a given digit was from a different category than the previous digit (e.g., “4” being in the “low” category followed by a “9” being in the “high” category). If two consecutive digits were from the same category (e.g., “6” followed by “8,” both being in the “high” category), the presentation was labeled as a non-switch presentation. Since accuracy data were not collected until the end of each string of digits, switch and non-switch trials were only intended to be analyzed for RT on each individual trial, not accuracy. Strings were presented in two manners: low-switch and high-switch. A “lowswitch” string was a series of presentations that contained no more than 25% category switches from one-digit presentation to the next and a “high switch” string was one that contained at least 50% or more category switches among the presentations. This was done in order to avoid chance performance settling at 50% with a binomial variable. The low-switch and high-switch categorizations were used simply as a control measure and therefore were not intended to be analyzed as independent variables. A total of 4 blocks were presented (two in quiet and two in MTB). Each block contained the four string presentations (i.e., switch condition x noise condition).
Effort Rating: Following each block for both the quiet and MTB condition, participants were asked to rate their perceived effort on a visual scale ranging from 0 (no effort) to 10 (most effort) by typing the corresponding value on the keyboard.
RESULTS
Results for the AS experiment were analyzed using a paired-samples t-test to examine accuracy results and a within-subjects repeated-measures 2 (condition: quiet vs. noise)×2 (task condition: switch vs. non-switch) factorial ANOVA to examine RT results.
A paired-samples t-test was conducted to examine the effect of noise condition on digit recall accuracy. Digit recall accuracy scores (proportion correct) were significantly higher for the quiet condition (M=0.88, SE=0.024) compared to the noise condition (M=0.64, SE=0.037.), t(15)=5.03, p <0.001, d=1.41.
A repeated-measures factorial ANOVA conducted on RT revealed a significant main effect of noise condition; the mean RTs for the quiet condition (M=1,671 ms, SE=101.6) were significantly faster than the noise condition (M=1,997 ms, SE= 124.8), F(1,60)=4.39, p=0.04, ηp2 =0.07. A significant main effect of task condition was also observed; mean RTs of the non-switch presentations (M=1,641 ms, SE=91.9) were significantly faster than the switch presentations (M =2,027 ms, SE=129.5), F(1,60)=6.14, p=0.016, ηp2 =0.09. Aggregate data are displayed in Figure 2. There was no significant interaction, F(1, 60)=0.021, p=0.88. As a follow-up analysis, a one-way repeated-measures ANOVA was performed on mean RTs of switch and non-switch presentations in quiet and noise. Results showed a significant omnibus test, F(3, 45)=16.02, p < 0.001. Pair-wise comparisons showed that mean Noise/Switch presentation RTs (M=2,178 ms, SE=199.5) were significantly longer than all other conditions. No other pair-wise comparisons were significant (p>0.05).
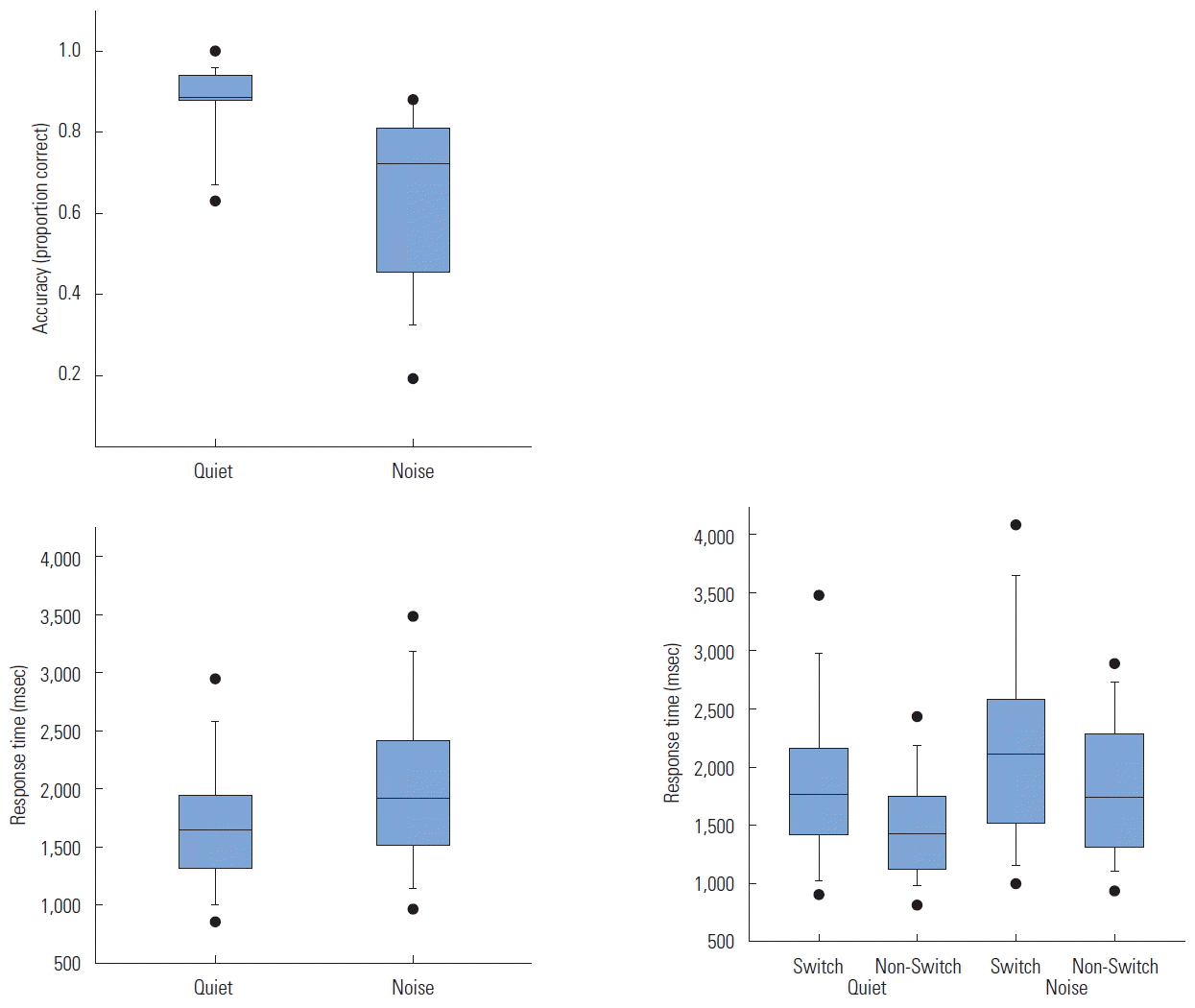
Results from the AS task are shown in box-plot form. In the upper panel, digit recall accuracy per noise condition is shown. In the lower left panel response time per noise condition is shown, and in the lower right panel, response time per trial condition (switch/non-switch) and noise condition is shown.
After each block, listeners rated their perceived effort using a rating scale from 0–10. A Mann-Whitney U test was used to analyze subjective effort rating in quiet and noise conditions. For the AS task, the test results revealed significantly less effort in quiet (median=4.00) than in noise (median=5.94), U= 54.5, z=−2.77, p<0.01, r=−0.49 (see Figure 3).
DISCUSSION
The results of Experiment 1 demonstrate that adding MTB to the AS task significantly impacted both accuracy and RT. Performance accuracy was significantly poorer for conditions with MTB present, and the RT data show that responses were also significantly slower in the noise condition. These findings show that the addition of noise, even at relatively low levels (90% SNR), significantly affects attention-related processing abilities, presumably as a result of requiring more cognitive resources to perform the tasks in an adverse listening environment. A reasonable interpretation of this decrease in performance is that it is due to an increase in listening effort [40]. This explanation is further substantiated by participants’ subjective listening effort ratings which showed significantly higher effort when the MTB was added. RTs were also significantly higher when the digit presentations were of the switch category compared to the non-switch category. This finding demonstrates that performing a mental switch from one category to the other creates a temporary increase in attentional load, and that increase is measurable using RTs.
During the task, the participants’ attentional focus was likely directed toward the memory bank of the previous trial [31,47]. A presentation of a digit in the same memory bank simply requires an update. A subsequent presentation of a digit in the other category requires a shift in attentional focus to the other memory bank followed by an update. This indicates that as participants updated and maintained a running total of information in two separate memory banks, the information took longer to process when the participants were required to switch from one bank to the other, rather than continually updating the same bank. Since only one digit was presented per trial, the act of mentally switching between categories increased the cognitive load on the participant. Thus, for switch presentations, the increased amount of time it took for participants to switch from one category to the other can be reflective of the increase in mental effort [47]. In this experiment, the Noise/Switch condition was designed to be the most difficult (and, thus, effortful), consisting of both an adverse listening environment as well as the increased cognitive load of switching categories. Therefore, the finding that this particular condition had significantly longer RTs than all other conditions is consistent with findings from other studies using similar task demands, with this having the added requirement of specific attentional control.
Experiment 2: Working memory span task
The ability to assess the semantic plausibility, or truth value, of sentences as well as to remember digits spoken both in quiet or MTB was tested using a complex working memory span task. Listeners were asked to determine whether a sentence was true or false while simultaneously remembering digits presented prior to each sentence. By assessing two different sentence types as well as the ability to store digit information while simultaneously recalling the truth value of each sentence, the task assesses how a significant cognitive load can affect one’s accuracy and RT.
Procedure: Digit span and SNR assessment
Digit span
Each participant’s digit span was measured in quiet to determine the number of sentences to be presented in the WMST experiment. The digit span task is a classic measure of short term memory with well-established validity and reliability [50]. A list of digits (0–9) was presented at 60 dB SPL via loudspeaker, with a 500 ms gap between presentations. Starting with a string of three digits, the participant was instructed to repeat back the digit sequence. Each sequence length was presented a total of three times. Based on a 2-down 1-up procedure, the string length increased by one digit if two of the three sequences were correctly recalled, indicating 70.7% accuracy criteria. This process continued until the participant could no longer repeat back at least two of the three strings correctly. The participant’s span was therefore considered the longest digit sequence repeated back correctly two out of three times. The result of this task was then used to establish the number of sentences presented in the WMST experiment. Figure 4 depicts the experimental procedure for Experiment 2 (see Table 1).

A schematic representation of the WMST experimental set up is depicted. A digit and a sentence, separated by a 100 ms gap, were presented through a speaker in both quiet and in the presence of MTB. The RT (ms) was a measure of how quickly the participant determined the truth value of the sentence. Following this keystroke, there was a 100 ms gap before the cycle repeated.
SNR assessment
The method for determining each participant’s 90% SNR was identical to Experiment 1 except sentences were used instead of digits. This level was then used as the setting for the noise condition in Experiment 2 (see Table 1).
Working memory span task
In this task, participants were presented with a sequence of digits and SVO and OR true/false sentences. First, a digit was presented followed by a 100 ms gap and then a sentence. After each sentence, the participant responded by pressing a key indicating whether the sentence was true or false. This response was measured as the participant’s sentence accuracy. The time between the end of the sentence and the response was recorded as the RT. One hundred ms after the participant’s response, the next digit was presented. After the sequence of digits and sentences was complete, the participant was instructed to recite all of the digits presented in serial order; the participant needed to recall each digit in its correct serial position in order for the sequence to be counted as correct. This was recorded as the participant’s digit accuracy.
To maximize the probability that participants were listening until the end of every sentence, dummy sentences were also included in each block. The dummy sentences were created in such a way that their truth value could not be determined until the last word in the sentence was presented (e.g., SVO Dummy: “The dog found the boy by the woods in the sky,” and OR dummy: “The boy that the dog found in the woods was plastic”). This ensured that participants were listening to each sentence in its entirety before determining its truth value, and were subsequently limited in their ability to rehearse digits during sentence presentations. Dummy sentences were recorded by the same male speaker as other sentence stimuli. In total, 4 noise blocks (2 SVO, 2 OR) and 4 quiet blocks (2 SVO, 2 OR) were presented. To impose sufficient cognitive load, the number of sentences presented in each block was equal to each participant’s digit span + 1, which was informed by pilot data. Each block contained an equal number of true and false sentences and two dummy sentences. In cases where the subject’s digit span was an odd number, each block contained an additional true sentence.
Effort Rating: Following each condition, participants were asked to rate their perceived effort. This effort scale ranged from 0 (no effort) to 10 (most effort).
RESULTS
Results for the WMST experiment were analyzed using a paired-samples t-test and two within-subjects repeated-measures 2 (condition: quiet vs. noise)×2 (complexity: SVO vs. OR) factorial ANOVAs. The dependent variables in this experiment were digit recall accuracy, sentence verification accuracy, and sentence response time. The independent variables were noise condition (quiet vs. noise) and sentence complexity (SVO vs. OR). A paired-samples t-test was conducted to examine the effect of noise condition on digit recall accuracy. Digit recall accuracy scores (proportion correct) were significantly higher for the quiet condition (M=0.67, SE=0.067) compared to the noise condition (M=0.51, SE=0.058), t(15)= 2.40, p =0.03, d =0.64. The ANOVA conducted on sentence verification accuracy showed no main effect of noise condition; the quiet condition (M=0.82, SE=0.026) was not different than the noise condition (M=0.78, SE=0.028), F(1,60)= 1.57, p=0.22. There was a significant main effect of sentence complexity; the overall sentence accuracy was significantly better for SVO condition (M=0.87, SE=0.019) than OR condition (M=0.73, SE=0.027), F(1,60)=17.0, p <0.001, ηp2 =0.22. There was no significant interaction, F(1, 60)=0.22, p =0.64. Figure 5 presents sentence accuracy results across conditions and complexity. The ANOVA conducted on RT showed no main effect of noise condition; the quiet condition (M=1,208 ms, SE=94.1) was not different than the noise condition (M= 1,174 ms, SE=82.5), F(1,60)=0.41, p=0.84. There was also no main effect of sentence complexity; RTs were not significantly different between SVO (M=1,148 ms, SE=88.8) and OR conditions (1,234 ms, SE=88.0), F(1,60)=0.26, p=0.61. There was no significant interaction, F(1,60)=0.49, p=0.49.
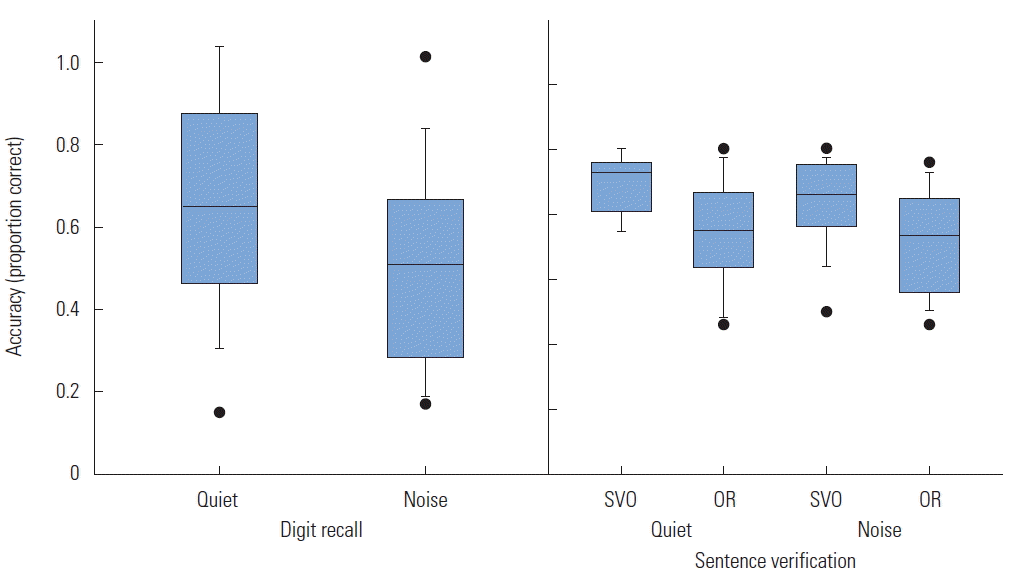
Results of the WMST are displayed in box-plot form. Digit recall accuracy per noise condition is shown in the left panel and sentence judgment accuracy per trial condition (SVO/OR) and noise condition is shown in the right panel.
After each trial, listeners rated their perceived effort using a rating scale from 0–10. A Mann-Whitney U test was used to analyze subjective effort rating in quiet and noise conditions. For the WMST, there was no significant difference in subjective effort between quiet (median =5.31) and noise (median=5.19), U=121.5, p=0.81, r=−0.04 (see Figure 3).
DISCUSSION
Like Experiment 1, the results of Experiment 2 also showed that the primary experimental measurement, in this case digit recall accuracy, was significantly poorer when MTB noise was present, indicating that background noise affected the participants’ ability to store, process, and maintain information in adverse listening conditions. The complexity of the sentences affected the participants’ ability to judge the truth value of the sentences, with the more complex OR sentences having significantly lower accuracy scores than the simpler SVO sentences. This finding likely reflects the increased language processing required for the OR sentences. The addition of noise had no effect on RT in Experiment 2. It is possible that in terms of sentence complexity, the participants were trading off accuracy performance with speed performance; accuracy for the more difficult OR sentences was significantly lower than SVO, but RTs were the same. Therefore, participants were taking the same amount of time to process the easier SVOs and more difficult ORs, which resulted in lower accuracy for the OR sentences. This performance tradeoff could also provide a possible explanation as to why the digit recall accuracy was better in quiet than in noise, but no difference in RTs between the quiet and noise conditions. Participants spent the same amount of time processing sentences in both conditions, but had better digit recall performance in quiet. With the noise condition designed to be the more difficult listening condition, the participants could have been trading off better digit recall performance with faster RT performance. Similarly, there was no significant difference in subjective effort ratings between the quiet and noise conditions. The participants taking the same amount of time to respond in both the quiet and the noise conditions could also contribute to the subjective effort ratings being the same for both conditions in the WMST.
General discussion
The goal of this study was to assess WM performance and the effects of a MTB interferer while completing novel attention-controlled, auditory-based cognitive tasks. The results of both experiments demonstrated that the presence of MTB significantly reduced the listeners’ ability to perform both auditory cognitive tasks. In Experiment 1, listeners’ ability to rapidly switch their attention was measured using an updating task paradigm. In order for participants to achieve high levels of accuracy with short RTs, careful control of attention was required. Adding noise significantly decreased accuracy and increased RTs. In addition, updating during switch trials was significantly longer than non-switch trials for both quiet and noise conditions. The switch cost, which represents the average RT difference between the switch and non-switch conditions, is the purest measure in this study of the time required to shift attention from one item to the next. As such, the significant cost measured in this experiment demonstrates the attentional load in these presentations. These results suggest that the switching of attention is significantly affected in the presence of noise due to an increase in cognitive load.
In Experiment 2, a complex WMST was constructed to measure serial digit recall along with basic language processing. The results indicated that listeners’ overall performance was poorer in recalling digits in the adverse listening condition; listeners were able to recall fewer digits in the presence of MTB. As hypothesized, not only was sentence verification accuracy significantly higher for SVO sentences than for OR sentences, but overall sentence verification accuracy was not different when MTB was added. Sentence verification depends heavily on intelligibility; in order to be able to determine the truth value of the sentences, participants must first be able to correctly identify all words in the sentence. Since the level of the background noise was intended to keep intelligibility levels high (90%), the finding that the addition of MTB had no effect on sentence verification demonstrates that intelligibility could be maintained throughout the experiment.
At its core, the WMST is a span task. Additional loads were added to allow a more comprehensive assessment of the WM system, including attentional control. A high accuracy score for sentence verification helps assure that the language processing served as a sufficient processing load during the task. Making decisions on the semantic plausibility of sentences requires controlled attention and language processing, which served to limit active digit rehearsal. Therefore, sentence verification accuracy can be thought of as a quality-control measure for the WMST digit recall accuracy, positioned to sufficiently tax WM cognitive resources. Thus, lower digit recall accuracy performance in the presence of background noise is likely attributed to an increase in cognitive processing load during the sentence verification portion of the task, not decreased intelligibility of the digits.
When MTB was added, overall performance dropped. Two possibilities have merit. First, the presence of an interfering sound that has many characteristics of speech posed a distractive load to attentional maintenance. This argument might explain the greater sensitivity of the AS task given its heightened attentional requirements. Second, even though intelligibility was impacted minimally, the redundant cues in speech may have been reduced, requiring greater top-down resources to achieve comprehension. In the ELU model [17], for instance, as implicit processing breaks down due to an impoverished input signal, explicit processing occurs which requires substantially increased resources for comprehension, thus reducing resources for other tasks. This effect has been demonstrated in experiments where levels of noise are low enough that intelligibility remains at near-ceiling level [41]. The first argument can be applied to a greater degree for Experiment 1, given the increased attentional requirements of the AS task. Likewise, the second argument applies to both experiments and also fits within the ELU framework given that all of the stimuli relevant to the tasks were language-based, and may be more applicable to the WMST considering the high degree of language processing involved in the task. Further study may illuminate the relative contributions of these factors.
The addition of MTB in Experiment 1 had a detrimental effect on both accuracy performance and RTs. This suggests that the switching of attention is negatively impacted by background noise, a notion that is corroborated by subjective effort ratings being significantly higher in the MTB condition compared to the quiet condition. The low level of background noise was chosen so as not to interfere with intelligibility of the stimuli, but instead was meant to tax cognitive resources. Participants rated the task as requiring significantly more effort in the noise condition. Consistently switching attention back and forth between two memory banks while also ignoring background noise requires more cognitive resources than switching attention in quiet. Decreased accuracy and increased RTs combined with the finding that subjective effort ratings were also affected by MTB shows that the addition of noise to cognitive tasks that require the consistent switching of attention are more effortful in the presence of modulated background noise. The participants’ perception of increased effort was substantiated by a drop in performance.
The addition of MTB in Experiment 2 had no effect on sentence verification accuracy, nor did participants subjectively rate the noise condition as being more difficult. The WMST consists of two concurrent sub-tasks: a span and language comprehension interdigitated within the span. Given that the sentences were readily intelligible even in noise, and that there was no carry-over (i.e., once a decision was made about a sentence, all information related to the sentence could be forgotten), this component of the WMST likely did not require substantial perceived effort. However, the span component of the WMST was likely perceived as challenging. First, there was significant time between presentations of the span elements in which rehearsal was largely prevented. Second, the block length was set to one element greater than the listener’s digit span. The instructions to the listener did not differentiate between the subtasks within the WMST. As such, a listener may have responded to the effort he or she expended for only the span component, the language processing, or a holistic impression of total effort. Accordingly, the likely outcome over a group of participants is one that would have an increased variability with an unpredictable distribution. Figure 3 supports the notion that at least some of the participants may have been responding to individual aspects of the WMST rather than the perceived effort over the grand sum of the task. Therefore, a conclusion regarding the perceived effort change with MTB in the WMST cannot be reliably made.
The results from this study support models of WM that describe attention as a separate control mechanism for WM. Participants were required to either maintain fixed attentional focus or shift their attention according to the presentation. Both tasks used in this study demand specific attentional control; the WMST involves shifting between the span element and the language processing, whereas the AS task has a relatively greater attentional requirement of shifting between bins that need updating on a more frequent basis as well as having a minimal processing and memory load. According to Cowan’s theoretical framework, the maintenance of the items (the memory span in the WMST and the size of each bin in the AS task) in short term memory is a result of activation, which is understood to be the mechanism of attention [28]. In the WMST, the shift from attending and updating the span to processing the sentence results in a decay of the now unattended span [28].
As described, the AS task has an augmented attentional load which distinguishes it from many other tasks used in published literature. Attentional resources have been described as a central bottleneck limiting retrieval to a single item at a time [33]. Thus, switching between bins rapidly is required to complete the AS task. The extent to which this bottleneck acts as such differs from person to person, and therefore can be used to differentiate individual WM performance.
The results of the AS task are robust, more so than many tasks that include concurrent tasks such as dual-tasks or our WMST. A power analysis indicated that the minimum number of subjects is just a single subject for the AS task, and 12 for the WMST for a power of 0.8. The primary differentiator between the AS and WMST is that the direct measure of attention switching as a substrate of attentional control. A reasonable conclusion is that a task that has high attentional control requirements, such as the AS task, relative to storage and processing may allow a greater sensitivity than other traditional tasks. Indeed, this has been the case with a similar task in other modalities [45,46].
Current thinking on the WM system describes a modality-specific peripheral store and a general central mechanism [36]. Their relative contributions to WM are not fully understood, but it is known that attention is a part of the central mechanism. Consequently, differentiators of central and peripheral components are required to understand the mechanisms responsible for WM performance. As such, tasks that can specifically tap the attentional resource have the additional advantage of potentially serving as this differentiator. Based on the findings of the current experiments, the addition of background noise impacts the efficiency of attentional control mechanisms. If the goal is to differentiate performance of central and peripheral components of WM in the auditory modality, a task that has minimal attentional requirements may be the most effective in delineating the relative contribution of the peripheral component.
Overall, the present study builds on the previous findings in auditory WM [38–40]. Unlike traditional dual-tasks where attention is alternated between two different modalities, the present study relies on the auditory modality only. A significant advantage of an auditory-based cognitive task is that it can be used not only to measure a participant’s listening effort objectively, but can also be used as a measure of language comprehension. The processing portion of the complex WM task in this study uses sentence verification rather than simply repeating sentences in a speech recognition task. Verification requires an additional level of processing compared to recognition. In a task requiring sentences to be repeated, processing of the sentence ends once the participant has spoken the words. However, in a verification task, sentence recognition is only the first step in the process; deciding whether the sentence is true or false cannot be completed until additional mental steps have taken place, thus illustrating the increased processing requirements necessary for a verification task. Therefore, sentence verification accuracy is a more relevant measure of speech comprehension than speech recognition cognitive tasks when attempting to measure listening effort. A second advantage designing robust tasks to assess auditory WM is that interpretations can be more easily attributed to mechanisms within the auditory modality. These experiments showed that with careful integration of attentional control, especially in the AS tasks, these tools can be used to further ascertain the contribution of the central vs. peripheral mechanisms [36].
While the findings from this study are demonstrative, they were limited in scope. For example, the study allowed elucidation of the importance of attentional mechanisms and the relative impact of MTB, but not in a manner that is applicable to clinical populations at this time. Given the degraded signal output from impaired peripheral auditory systems, we suspect that equivalent conditions would result in a greater tax to WM and/or attentional mechanisms reducing overall performance for the hearing impaired. Stated in terms of the ELU model, the degraded cochlear output would increase the number of instances the explicit loop would be required for comprehension. Another limitation is that the study design limited the degree to which associations could be made with listening effort. Admittedly, this association was a secondary goal, but one that could prove interesting in how attention relates to perceived effort. Moreover, the perceived effort measurements in the WMST did not allow differentiation of which component of that task accounted for the perceived effort rating, thus limiting interpretation. Finally, while the use of MTB was based on pilot data, the impacts of other types of distractors and/or noises may be useful to understand, especially in how they relate to real-world situations.
Both of these tasks were designed to tax cognitive resources thought to be crucial for speech understanding. The complex WMST and AS tasks used in the current study required listeners to store and process information concurrently under attentional control; this is a skill highly utilized in conversations and general communication. In this study, adding MTB increased the load on central resources resulting in significantly increased time needed to process information and also reducing the accuracy of temporarily stored speech information. Since speech intelligibility remained unaffected during the tasks, the resulting decrease in performance can be attributed to an increase in cognitive load. The findings of this study demonstrate that increasing the processing load in WM by adding MTB negatively impacts listening effort but not speech understanding, and that a high-attentional load provides a sensitive measure of WM as well as offering a potential method to delineate between peripheral and central WM mechanisms.